Murua, Alejandro
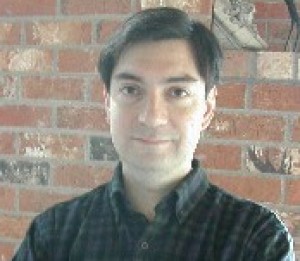
- Full Professor
-
Faculty of Arts and Science - Department of Mathematics and Statistics
André-Aisenstadt Office 4221
Courriels
Affiliations
- Membre Centre de recherches mathématiques
- Membre CRM Centre de recherches mathématiques
- Membre Institut de valorisation des données
- Membre IVADO Institut de valorisation des données
Research area
- Computational statistics
- Bayesian statistics
- Statistical learning algorithm
- Complex data exploration in higher dimensions
- Object identification
- Gibbs and Markov fields
Student supervision Expand all Collapse all
Abstract
Abstract
Abstract
Abstract
Abstract
Abstract
Abstract
Abstract
Abstract
Research projects Expand all Collapse all
Centre de recherches mathématiques (CRM) FRQNT/Fonds de recherche du Québec - Nature et technologies (FQRNT) / 2022 - 2029
Brain connectivity-based optimization of non-invasive brain stimulation to improve cognitive symptoms in schizophrenia IRSC/Instituts de recherche en santé du Canada / 2019 - 2026
Bayesian deeplearning prediction with sparse graphs CRSNG/Conseil de recherches en sciences naturelles et génie du Canada (CRSNG) / 2019 - 2025
Investment portfolio design and optimal execution of automated trading strategies: An exploratory research program MITACS Inc. / 2019 - 2019
Gibbs-repulsion and determinantal processes for statistical learning SPIIE/Secrétariat des programmes interorganismes à lintention des établissements / 2018 - 2020
Uplift Models Extension for Smart Marketing. MITACS Inc. / 2017 - 2018
Variable Selection for Uplift Modeling. MITACS Inc. / 2017 - 2017
CENTRE DE RECHERCHES MATHEMATIQUES (CRM) FRQNT/Fonds de recherche du Québec - Nature et technologies (FQRNT) / 2015 - 2023
MODELE DE MELANGE AVEC NOYAUX POUR LA CLASSIFICATION DES DONNEES DE GRANDE DIMENSION Innovation, Sciences et Développement économique Canada / 2014 - 2015
KERNEL-BASED NON-PARAMETRIC BAYESIAN CLUSTERING MODELS CRSNG/Conseil de recherches en sciences naturelles et génie du Canada (CRSNG) / 2013 - 2019
INNOVATIVE CHEMOGENOMIC TOOLS TO IMPROVE OUTCOME IN ACUTE MYELOID LEUKEMIA Génome Canada / 2013 - 2017
INNOVATIVE CHEMOGENOMIC TOOLS TO IMPROVE OUTCOME IN IN ACUTE MYELOID LEUKEMIA Génome Québec / 2013 - 2017
COMPUTATIONAL RESOURCES FOR RESEARCH IN MATHEMATICS AND STATISTICS CRSNG/Conseil de recherches en sciences naturelles et génie du Canada (CRSNG) / 2013 - 2015
Selected publications Expand all Collapse all
The penalized biclustering model and related algorithms
The conditional-Potts clustering model
The Gibbs-plaid biclustering model
Kernel-based mixture models for classification
The conditional-Potts clustering model
On Potts model clustering, kernel $K$-means, and density estimation
Model based document classification and clustering
Functional connectivity mapping using the ferromagnetic Potts spin model
Country Clustering to Evaluate Global Health Outcomes
Mapping Functional Connectivity Using Potts Spin Model. Proceedings of the 14th Scientific Meeting of the International Society for Magnetic Resonance in Medicine
Probabilistic segmentation and intensity estimation for microarray images
Resting State Connectivity of Anterior and Posterior Cingulate Corteces Using Potts Spin Model. Proceedings of the 14th Scientific Meeting of the International Society for Magnetic Resonance in Medicine
On Potts model clustering, kernel K-means and density estimation.
Clustering fMRI time series in the wavelet domain. Proceedings of the 13th Scientific Meeting of the International Society for Magnetic Resonance in Medicine
Optimal transformations for prediction in continuous-time stochastic processes: finite past and future
Estimation and consistency for linear functionals of continuous-time processes from finite data set, II: Optimal Transformations for Prediction.
Hierarchical model-based clustering of large datasets through fractionation and refractionation
Assessment and Pruning of Hierarchical Model Based Clustering. The Ninth International Conference on Knowledge Discovery and Data Mining
Upper bounds for error rates associated to linear combination of classifiers
Hierarchical model-based clustering of large datasets through fractionation and refractionation. The Eighth International Conference on Knowledge Discovery and Data Mining
Evaluation of sequential importance sampling for blind deconvolution via a simulation study. Proceedings of the XI European Signal Processing Conference
Model-Based Clustering and Data Transformations for Gene Expression Data
Speech recognition using randomized relational decision tree
A 2D extended HMM for speech recognition. Proceedings of the IEEE International Conference on Acoustics, Speech, and Signal Processing
On the regularity of spectral densities of continuous-time completely linearly regular processes
Optimal transformations for prediction in continuous time stochastic processes.
Estimation and consistency for linear functionals of continuous-time processes from a finite data set, I: Linear Predictors.
Stop consonants discrimination and clustering using nonlinear transformations and wavelets.
Classification and clustering of stop consonants via nonparametric transformations and wavelets. Proceedings of the IEEE International Conference on Acoustics, Speech, and Signal Processing
Existence and multiplicity of solutions with prescribed period for a second order quasilinear ODE
On the number of $2\pi$ periodic solutions for $u''+g(u)=s(1+h(t))$ using the Poincaré-Birkhoff theorem