Maire, Florian
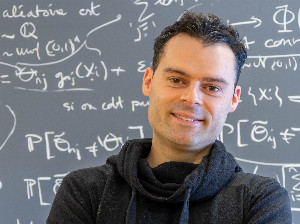
- Associate Professor
-
Faculty of Arts and Science - Department of Mathematics and Statistics
André-Aisenstadt Office 4253
Courriels
Courses
- MAT1978 H - Probabilités et statistique
Research area
- Algorithm optimisation
- Computational statistics
- Bayesian statistics
- Applied probability
- Markov chain
- Statistical learning algorithm
- Gibbs and Markov fields
Student supervision Expand all Collapse all
Efficacité de l'algorithme EM en ligne pour des modèles statistiques complexes dans le contexte des données massives
Theses and supervised dissertations / 2020-11
Martel, Yannick
Abstract
Abstract
The EM algorithm Dempster et al. (1977) yields a sequence of estimators that converges to the maximum likelihood estimator for missing data models whose maximum likelihood estimator is not directly tractable. The EM algorithm is remarkable given its numerous applications in statistical learning. However, it may suffer from its computational cost. Cappé and Moulines (2009) proposed an online version of the algorithm in models whose likelihood belongs to the exponential family that provides an upgrade in computational efficiency in large data sets. However, the conditional expected value of the sufficient statistic is often intractable for complex models and/or when the missing data is of a high dimension. In those cases, it is replaced by an estimator. Many questions then arise naturally: do the convergence results pertaining to the initial estimator hold when the expected value is substituted by an estimator? In particular, does the asymptotic normality property remain in this case? How does the variance of the estimator of the expected value affect the asymptotic variance of the EM estimator? Are Monte-Carlo and MCMC estimators suitable in this situation? Could variance reduction tools such as control variates provide variance relief? These questions will be tackled by the means of examples containing latent data models. This master’s thesis’ main contributions are the presentation of a unified framework for stochastic approximation EM algorithms, an illustration of the impact that the estimation of the conditional expected value has on the variance and the introduction of online EM algorithms which reduce the additional variance stemming from the estimation of the conditional expected value.
Étude d'algorithmes de simulation par chaînes de Markov non réversibles
Theses and supervised dissertations / 2020-10
Huguet, Guillaume
Abstract
Abstract
Markov chain Monte Carlo (MCMC) methods commonly use chains that respect the detailed
balance condition. These chains are called reversible. Most of the theory developed for
MCMC evolves around those particular chains. Peskun (1973) and Tierney (1998) provided
useful theorems on the ordering of the asymptotic variances for two estimators produced by
two different reversible chains.
In this thesis, we are interested in non-reversible chains, which are chains that don’t
respect the detailed balance condition. We present algorithms that simulate non-reversible
chains, mainly the Guided Random Walk (GRW) by Gustafson (1998) and the Discrete
Bouncy Particle Sampler (DBPS) by Sherlock and Thiery (2017). For both algorithms, we
compare the asymptotic variance of estimators with the ones produced by the Metropolis-
Hastings algorithm.
We present a recent theoretical framework introduced by Andrieu and Livingstone (2019)
and their analysis of the GRW. We then show that the DBPS is part of this framework
and present an analysis on the asymptotic variance of estimators. Their main theorem
can provide an ordering of the asymptotic variances of two estimators resulting from nonreversible
chains. We show that an estimator could have a lower asymptotic variance by
adding propositions to the DBPS. We then present empirical results of a modified DBPS.
Through the thesis we will mostly be interested in chains that are produced by deterministic
proposals. We show a general construction of the delayed rejection algorithm using
deterministic proposals and one possible equivalent for non-reversible chains.