Gagnon, Philippe
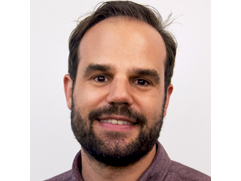
- Assistant Professor
-
Faculty of Arts and Science - Department of Mathematics and Statistics
André-Aisenstadt
Courriels
Research area
- Markov chain Monte Carlo methods
- Regression methods
- Robust statistics
- Model/variable selection
- Bayesian statistics
My research interests are Bayesian robustness against outliers and Markov chain Monte Carlo methods. Recently, I introduced methodology for robust model/variable selection and parameter estimation in linear regression, along with efficient algorithms for achieving these tasks. My research has so far been more on the theoretical/methodological side. My objective for the next years is to apply the tools I developed in actuarial science, and to introduce new ones of interest for actuaries, such as Bayesian robust generalized linear models. In particular, I want to develop an automatic data analysis procedure in which the models are robust and trained through a full and exact Bayesian analysis. See my personal web page for more details and a list of my publications.
Advertisement: I am looking for students with a strong background in either: theoretical statistics, applied statistics and actuarial science, or computer science (the plan is also to develop R packages for a user-friendly and efficient implementation of the methods). There exist multiple funding opportunities. In particular, I recently obtained grants that are dedicated to the funding of world-class M.Sc. and Ph.D. students, and postdoctoral researchers (see my personal web page for more details). Also, prospective students and postdocs can apply for scholarships such as those of NSERC and FRQNT. Please do not hesitate to contact me if you are interested. I aim to provide an environment to my students in actuarial science and statistics that is equitable, inclusive and diversified. In particular, recruiting will be done according to Université de Montréal's Equity, Diversity and Inclusion policy.
Student supervision Expand all Collapse all
Sélection de modèles robuste : régression linéaire et algorithme à sauts réversibles
Theses and supervised dissertations / 2017-10
Gagnon, Philippe
Abstract
Abstract
Model selection and parameter estimation are two main aspects of statistical analysis. This thesis discusses these aspects from a Bayesian point of view via three papers. The first one deals with a computational procedure, named the reversible jump algorithm, that allows to simultaneously select models and estimate parameters. This sampler being difficult to tune in practice, we aim at providing guidelines to users for an optimal implementation. An optimally tuned sampler corresponds to a sampler that generates Markov chains that optimally explore their state space. Our goal is achieved through the optimisation of a stochastic process that corresponds to the limit (in distribution) of the sequence of stochastic processes engendered by the algorithm. In the second paper, a strategy leading to robust estimation of the parameters of a linear regression model in presence of outliers is presented. The strategy is to make assumptions that are more adapted to the eventual presence of outliers, compared with the traditional model assuming normality of errors.
This normality assumption is indeed replaced by a super heavy-tailed distribution assumption. Robustness, which is represented by the convergence of the posterior distribution of the parameters (based on the whole sample) towards that arising from the nonoutliers only, is guaranteed when the number of outliers does not exceed a given threshold. Finally, the results presented in the first two papers are combined to introduce a Bayesian robust principal component regression approach that involves model selection in the prediction process. The characteristics of this approach contribute to increase the accuracy of the predictions produced.