Craig, Morgan
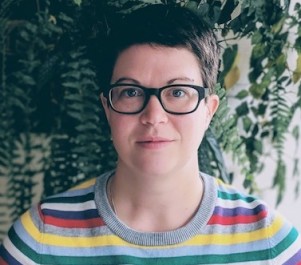
- Associate Professor
-
Faculty of Arts and Science - Department of Mathematics and Statistics
André-Aisenstadt Office 5243
Courriels
Affiliations
- Membre Centre de recherche du CHU Sainte-Justine
- Membre Centre for Applied Mathematics in Bioscience and Medicine (CAMBAM)
- Membre Groupe de recherche universitaire sur le médicament
- Membre GRUM Groupe de recherche universitaire sur le médicament
- Membre Society for Industrial and Applied Mathematics
- Membre Society for Mathematical Biology
Courses
- MAT3450 A - Intro. modélisation math.
Research area
- Applied mathematics
- Modelisation
- Haematopoiesis
- Pharmacometrics
- Neutropenia
- Dynamical systems
- Delay differential equations
- Nonlinear dynamic
Dr. Morgan Craig is a Researcher at the Sainte-Justine University Hospital Research Centre and an Assistant Professor in the Department of Mathematics and Statistics at the Université de Montréal. She was a postdoc in the Department of Organismic and Evolutionary Biology at Harvard University working with Dr. Alison Hill after receiving her Ph.D. in Pharmaceutical Sciences from the Université de Montréal. The Quantitative and Translational Medicine Laboratory that she runs focuses on the application and implementation of quantitative approaches, particularly computational biology, to study how heterogeneity impacts on disease and treatment outcomes. Her research focuses on the development of predictive, mechanistic models in a variety of disease contexts, particularly cancers and diseases caused by viruses, to identify pathophysiological mechanisms and tailor therapeutic regimens according to patient-specific characteristics. Dr. Craig’s research is highly multidisciplinary and is conducted in close collaboration with experimentalists and clinicians.
Student supervision Expand all Collapse all
Abstract