Wolf, Guy
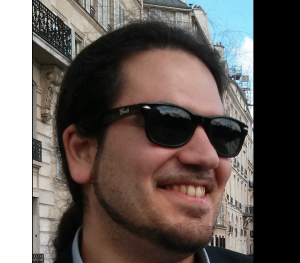
- Professeur agrégé
-
Faculté des arts et des sciences - Département de mathématiques et de statistique
André-Aisenstadt Local 6165
Courriels
Affiliations
- Membre Institut de valorisation des données
- Membre Institut des algorithmes d'apprentissage de Montréal
- Membre IVADO Institut de valorisation des données
- Membre MILA Institut des algorithmes d'apprentissage de Montréal
Cours donnés
- STT3795 H - Fond. théo. en sc. des données
Expertise
Mes sujets de recherche se situent à l'intersection de l'apprentissage automatique, des sciences des données et des mathématiques appliquées. En particulier, je suis intéressé par les méthodes d'exploration des données qui utilisent l'apprentissage des variétés et l'apprentissage profond géométrique. Je m'intéresse aussi aux applications d'analyse exploratoire des données biomédicales, surtout ceux qui portent sur les données de cellules uniques (p.ex. scRNA-seq et CyTOF).
Encadrement Tout déplier Tout replier
Horseshoe regularization for wavelet-based lensing inversion
Thèses et mémoires dirigés / 2024-03
Nafisi, Hasti
Abstract
Abstract
Gravitational lensing, a phenomenon in astronomy, occurs when the gravitational field of a massive object, such as a galaxy or a black hole, bends the path of light from a distant object behind it. This bending results in a distortion or magnification of the distant object's image, often seen as arcs or rings surrounding the foreground object.
The Starlet wavelet transform offers a robust approach to representing galaxy images sparsely. This technique breaks down an image into wavelet coefficients at various scales and orientations, effectively capturing both large-scale structures and fine details.
The Starlet wavelet transform offers a robust approach to representing galaxy images sparsely. This technique breaks down an image into wavelet coefficients at various scales and orientations, effectively capturing both large-scale structures and fine details.
The horseshoe prior has emerged as a highly effective Bayesian technique for promoting sparsity and regularization in statistical modeling. It aggressively shrinks negligible values while preserving important features, making it particularly useful in situations where the reconstruction of an original image from limited noisy observations is inherently challenging.
The main objective of this thesis is to apply sparse regularization techniques, particularly the horseshoe prior, to reconstruct the background source galaxy from gravitationally lensed images. By demonstrating the effectiveness of the horseshoe prior in this context, this thesis tackles the challenging inverse problem of reconstructing lensed galaxy images.
Our proposed methodology involves applying the horseshoe prior to the wavelet coefficients of lensed galaxy images. By exploiting the sparsity of the wavelet representation and the noise-suppressing behavior of the horseshoe prior, we achieve well-regularized reconstructions that reduce noise and artifacts while preserving structural details. Experiments conducted on simulated lensed galaxy images demonstrate lower mean squared error and higher structural similarity with the horseshoe prior compared to alternative methods, validating its efficacy as an efficient sparse modeling technique.